Learning analytics
<pageby nominor="false" comments="false"/>
Introduction
One could define learning analytics as collection of methods that allow teachers and maybe the learners to understand what is going on. I.e. all sorts of tools that allow to gain insight on participant's behaviors and productions (including discussion and reflections). The learning analytics community in their call for the 1st International Conference on learning analytics provides a more ambitious definition: “Learning analytics is the measurement, collection, analysis and reporting of data about learners and their contexts, for purposes of understanding and optimising learning and the environments in which it occurs”, i.e. also view it in a clearly transformative perspective.
See also:
- Wiki metrics, rubrics and collaboration tools
- Other articles in the Analytics category
- Visualization and social network analysis
The Society for Learning Analytics Research Open Learning Analytics (2011) proposal associates learning analytics with the kind of "big data" that are used in busines intelligence:
The rapid development of “big data” methods and tools coincides with new management and measurement processes in corporations. The term “business intelligence” is used to describe this intersection of data and insight. When applied to the education sector, analytics fall into two broad sectors (Table 1): learning and academic.
Learning analytics (LA) is the measurement, collection, analysis and reporting of data about learners and their contexts, for purposes of understanding and optimizing learning and the environments in which it occurs. Learning analytics are largely concerned with improving learner success.
Academic analytics is the improvement of organizational processes, workflows, resource allocation, and institutional measurement through the use of learner, academic, and institutional data. Academic analytics, akin to business analytics, are concerned with improving organizational effectiveness.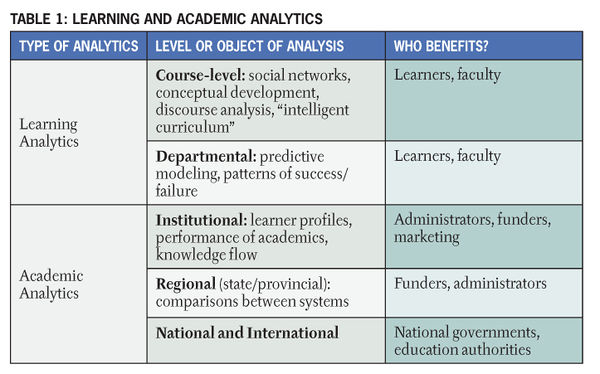
In other words, learning analytics concerns people concerned by teaching and learning, e.g. learners themselves, teachers, course designers, course evaluators, etc. Learning analytics is seen “LA as a means to provide stakeholders (learners, educators, administrators, and funders) with better information and deep insight into the factors within the learning process that contribute to learner success. Analytics serve to guide decision making about educational reform and learner-level intervention for at-risk students.” (Simons et al. 2011: 5)
In that sense, this definition is political and like many other constructs in the education sciences it promises better education. We therefore conclude the introduction that learning analytics either can be seen either (1) as tools that should be integrated into the learning environment and scenario with respect to specific teaching goals or (2) as a more general and bigger "framework" for doing "education intelligence". The latter also would include the former.
Frameworks
Some kinds of learning analytics have been known and used since education exists, like:
- Grades
- learning e-portfolios, i.e. students assemble productions and reflect upon these (use quite a lot in professional education)
- Tracking tools in learning management systems
- Cockpits and scaffolding used in many CSCL tools.
The question is where the locus of data collection and data analysis are and on how big a scale (number of students, activities and time) it is done. In radical constructivist thought, students should be self-regulators and the role of the system is just provide enough tools for them as individuals or groups for understanding what is going on. In more teacher-oriented socio-constructivism, the teachers, in addition, need indicators in order to monitor orchestrations (activities in pedagogical scenarios. In other designs such as direct instruction, the system should provide detail performance data that are rather based on quantitative test data.
The SoLAR strategy
The technical framework
This proposal addresses the need for integrated toolsets through the development of four specific tools and resources:
- A Learning analytics engine, a versatile framework for collecting and processing data with various analysis modules (depending on the environment)
- Adaptive content engine
- Intervention engine: recommendations, automated support
- Dashboard, reporting, and visualization tools
A short discussion
The proposal starts somehow with the assumption that education continues to use rather simple tools like courseware or somewhat now popular web 2.0 tools like personal learning environments. In other words, the fog is identifed as problem and not the way education is designed. I.e. this proposal focuses on "intelligence" as opposed to "design". If you like, it is more like "General motors" than "Apple". Related to that it is also assumed that "metrics" work, while in reality testing-crazy systems like the US high-school education has a much lower performance than design-based systems like the Finnish one.
The project proposes a general framework based on modern service-oriented architectures. So far, all attempts to use such architectures in education did fail, probably because of the lack of substantial long-term funding, e.g. see the e-framework project. We also a wonder a bit how such funding could be found, since not even the really simple IMS Learning Design has been implemented in a usable set of tools. In addition, even more simple stuff, like simple wiki tracking is not available, e.g. read wiki metrics, rubrics and collaboration tools
We would like to draw parallels with (1) the metadata community that spent a lot of time designing standards for describing documents and instead of working on how to create more expressive documents and understanding how people compose documents, (2) with business that spends energy on marketing and related business intelligence instead of designing better products, (3) with folks who believe in adaptive systems forgetting that learner control is central to deep learning and that most higher education is collaborative, (4) with the utter failure of intelligent tutoring systems trying to give control to the machine and (5) finally with the illusion of learning style. These negative remarks are not meant to say that this project should or must fail, but they are meant to state two things: The #1 issue is in education is not analytics, but designing good learning scenarios within appropriate learning environements (most are not). The #2 issue is massive long term funding. Such a system won't work before at least 50 man years over a 10 year period is spend.
Somewhat it also is assumed that teachers don't know what is going on and that learners can't keep track of their progress or more precisely that teachers can't design scenarios that will help both teachers and students knowing what is going on. We mostly share that assumption, but would like to point out that knowledge tools do exist, e.g. knowledge forum, but these are never used. This can be said in general of CSCL tools that usually include scaffolding and meta-reflective tools. In other words, this proposal seems to imply that that education and students will remain simple, but "enhanced" with both teacher and student cockpits that sort of rely on either fuzzy data minded from social tools (SNSs, forums, etc.) or quantitative data from learning mangement systems.
Finally, this project raises deep privacy and innovation issues. Since analytics can be used for assessment, there will be attemps to create life-long scores. In addition, if students are require to play the analytics game in order to improve chances, this will be other blow to creativity. If educational systems formally adopt analytics, it opens the way for keeping education in line with "main-stream" e-learning, an approach designed for training simple subject matters (basic facts and skills) through reading, quizzing and chatting. This, because analytics will work fairly easily with simple stuff, e.g. scores, lists of buttons clicked, number of blog and forum posts, etc.
Technology for supporting Collaborative learning
Soller, Martinez, Jermann and Muehlenbrock (2004, 2005:Abstract) develop a collaboration management cycle framework that distinguishs between mirroring systems, which display basic actions to collaborators, metacognitive tools, which represent the state of interaction via a set of key indicators, and coaching systems, which offer advice based on an interpretation of those indicators.
“The framework, or collaboration management cycle is represented by a feedback loop, in which the metacognitive or behavioral change resulting from each cycle is evaluated in the cycle that follows. Such feedback loops can be organized in hierarchies to describe behavior at different levels of granularity (e.g. operations, actions, and activities). The collaboration management cycle is defined by the four following phases” (Soller et al., 2004:6):
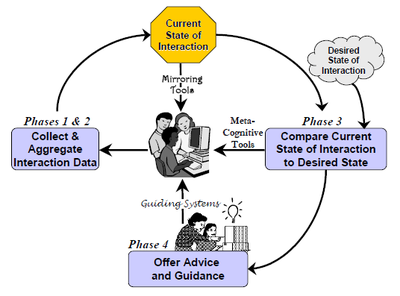
- Phase 1: The data collection phase involves observing and recording the interaction of users. Data are logged and stored for later processing.
- Phase 2: Higher-level variables, termed indicators are computer to represent the current state of interaction. For example, an agreement indicator might be derived by comparing the problem solving actions of two or more students, or a symmetry indicator might result from a comparison of participation indicators.
- Phase 3: The current state of interaction can then be compared to a desired model of interaction, i.e. a set of indicator values that describe productive and unproductive interaction states. “For instance, we might want learners to be verbose (i.e. to attain a high value on a verbosity indicator), to interact frequently (i.e. maintain a high value on a reciprocity indicator), and participate equally (i.e. to minimize the value on an asymmetry indicator).” (p. 7).
- Phase 4: Finally, remedial actions might be proposed by the system if there are discrepancies.
- Soller et al. (2005:) add a phase 5: “After exiting Phase 4, but before re-entering Phase 1 of the following collaboration management cycle, we pass through the evaluation phase. Here, we reconsider the question, “What is the final objective?”, and assess how well we have met our goals”. In other words, the "system" is analysed as a whole.
Depending on the locus and amount amount of computer-support, the authors then identify three kinds of systems:
- Mirroring tools automatically collect and aggregate data about the students’ interaction (phases 1 and 2 in Figure 1), and reflect this information back to the user with some kind of visualization. Locus of processing thus remains in the hand of learners or teachers.
- Metacognitive tools display information about what the desired interaction might look like alongside a visualization of the current state of indicators (phases 1, 2 and 3 in the Figure), i.e. offer some additional insight to both learners and teachers.
- Guiding systems perform all the phases in the collaboration management process and directly propose remedial actions to the learners. Details of the current state of interaction and the desired model may remain hidden.
Technologies
Since "Analytics" is a term that can include any kind of purpose as well as any kind of activity to observe and analyze, the set of technologies is rather huge and being developped in very different contexts. Recent interest in learning analytics seems to be sparked by the growing importance of on-line marketing (including search engine optimization) as well as user experience design of e-commerce sites. However, as we tried to show in our short discussion of CSCL technology, mining student "traces" has a longer tradition.
Software
According to Wikipedia, retrieved 14:06, 2 March 2012 (CET). “Much of the software that is currently used for learning analytics duplicates functionality of web analytics software, but applies it to learner interactions with content. Social network analysis tools are commonly used to map social connections and discussions”
None of the software listed comes even close to the SoLAR framework. We roughly can distinguish between software that gathers (and optional displays) data in very specific applications, software that mines the web and finally general purpose analysis/visualization software.
Ali, Hatala, Gašević & Jovanović (2011) as well as Asadi et al. (2011) provide a good overview of various learning analytics tools.
Social network analysis
Two popular ones are:
- SNAPP - “a software tool that allows users to visualize the network of interactions resulting from discussion forum posts and replies. The network visualisations of forum interactions provide an opportunity for teachers to rapidly identify patterns of user behaviour – at any stage of course progression. SNAPP has been developed to extract all user interactions from various commercial and open source learning management systems (LMS) such as BlackBoard (including the former WebCT), and Moodle. SNAPP is compatible for both Mac and PC users and operates in Internet Explorer, Firefox and Safari.”, retrieved 14:06, 2 March 2012 (CET).
- Pajek, is a program, for Windows, for analysis and visualization of large networks.
Tracking in e-learning platforms
- Teacher ADVisor (TADV): uses LCMS tracking data to extract student, group, or class models (Kobsa, Dimitrova, & Boyle, 2005).
- Student Inspector: analyzes and visualizes the log data of student activities in online learning environments in order to assist educators to learn more about their students (Zinn & Scheuer, 2007).
- CourseViz: extracts information from WebCT.
- Learner Interaction Monitoring System (LIMS): (Macfadyen & Sorenson, 2010)
LOCO Analyst
LOCO-Analyst “is implemented as an extension of Reload Content Packaging Editor, an open-source tool for creating courses compliant with the IMS Content Packaging (IMS CP) specification. By extending this tool with the functionalities of LOCO-Analyst, we have ensured that teachers effectively use the same tool for creating learning objects, receiving and viewing automatically generated feedback about their use, and modifying the learning objects accordingly.” (LOCO-Analyst, retrieved 14:06, 2 March 2012 (CET)).
Features:
- “feedback about individual student was divided into four tab panels: Forums, Chats, Learning, and Annotations [...]. The Forums and Chats panels show student’s online interactions with other students during the learning process. The Learning panel presents information on student’s interaction with learning content. Finally, the Annotations panel provides feedback associated with the annotations (notes, comments, tags) created or used by a student during the learning process.” (Ali et al. 2001)
- For each of the four "areas" key data can then be consulted.
Badges systems
- Educational badges systems that are managed through some kind of server technology can be used to harvest information about learner achievements, paths, skills, etc.
For example, “Mozilla's Open Badges project is working to solve that problem, making it easy for any organization or learning community to issue, earn and display badges across the web. The result: recognizing 21st century skills, unlocking career and educational opportunities, and helping learners everywhere level up in their life and work.” (About, retrieved 15:37, 14 March 2012 (CET).
Text analytics (data mining)
- TADA-Ed (Tool for Advanced Data Analysis in Education) combines different data visualization and mining methods in order to assist educators in detecting pedagogically important patterns in students’ assignments (Merceron & Yacef, 2005).
See also:
Cognitive tools with built-in Analytics
There are many cognitive tools, knowledge building environments, etc. allowing to co-create knowledge and that do include some information "on what is going on". Some are developed for education, others for various forms of knowledge communities, such as communities of practice
Examples:
- Cohere from Open University is a visual tool to create, connect and share Ideas.
- Knowledge forum and CSILE
- Wikis, see the Wiki metrics, rubrics and collaboration tools article
- concept map tools and other idea managers. Some of these run as services.
Social web-data-gathering and visualization software
Various general purpose tools could be used, such as:
See also:
- social bookmarking, reference manager and Citation index services. These also provide quite a lot of data on what authors and other users produce and organize.
Other tracking tools
- SAM (Student Activity Monitor)- “To increase self-reflection and awareness among students and for teachers, we developed a tool that allows analysis of student activities with different visualizations”. Could be used for Personal learning environments.
- Most research systems built in educational technology, do have built-in tracking technology, see for example the CoFFEE system
Data visualization software
Some online tools:
Locallly installed:
- OpenDX (not updated since 2007)
See also: Visualization and social network analysis
Links
Overviews and bibliographies
- 1st International Conference on learning analytics (includes a short introduction)
- Learning analytics - Wikipedia, the free encyclopedia
- Learning and Knowledge Analytics Bibliography by George Siemens (et al?).
Organizations and conferences
- Society for Learning Analytics Research (SoLAR), “on inter-disciplinary network of leading international researchers who are exploring the role and impact of analytics on teaching, learning, training and development.”
- International Educational Data Mining Society
- Educational Data Mining Conference
Web sites
- Learning and Knowledge Analytics, devoted to learning and knowledg. Managed by G. Simons.
Free online courses
- Introduction to Learning and Knowledge Analytics, An Open Online Course January 10-February 20, 2011. (course program, resources, and links)
- A Netvibes page made by G. Siemens pulls together various online productions.
Talks
- By Simon Buckingham Shum, jan 2012.
Various
- Awesome: DIY Data Tool Needlebase Now Available to Everyone By Marshall Kirkpatrick / November 30, 2010
Bibliography
We split it into two section with the idea that this entry may become separated some day.
Analytics
This bibliography is (so far) mostly based on:
- Penetrating the Fog (EduCause)
- George Siemens Learning & Knowledge Analytics Bibliography (Google Docs) based on a literature review by https://docs.google.com/document/d/1f1SScDIYJciTjziz8FHFWJD0LC9MtntHM_Cya4uoZZw/edit?hl=en&authkey=CO7b0ooK&pli=1
Entries
- Ali, L., Hatala, M. Gašević, D., Jovanović, J., "A Qualitative Evaluation of Evolution of a Learning Analytics Tool," Computers & Education, Vol. 58, No. 1, 2012, pp. 470-489 Abstract/HTML/ (Science direct)
- Arnold, K. E. (2010). Signals: Applying Academic Analytics, EDUCAUSE Quarterly 33(1). HTML
- M. Asadi, J. Jovanović, D. Gašević, M. Hatala (2011) A quantitative evaluation of LOCO-Analyst: A tool for raising educators’ awareness in online learning environments. Technical Report. [Online]. Available at https://files.semtech.athabascau.ca/public/TRs/TR-SemTech-12012011.pdf
- Baker, R.S.J.d., de Carvalho, A. M. J. A. (2008) Labeling Student Behavior Faster and More Precisely with Text Replays. Proceedings of the 1st International Conference on Educational Data Mining, 38-47.
- Baker, R.S.J.d., Yacef, K. (2009) The State of Educational Data Mining in 2009: A Review and Future Visions. Journal of Educational Data Mining, 1 (1), 3-17. PDF
- Baker, R.S.J.d. (2010) Mining Data for Student Models. In Nkmabou, R., Mizoguchi, R., & Bourdeau, J. (Eds.) Advances in Intelligent Tutoring Systems, pp. 323-338. Secaucus, NJ: Springer. PDF Reprint
- Baker, R.S.J.d. (2010) Data Mining for Education. In McGaw, B., Peterson, P., Baker, E. (Eds.) International Encyclopedia of Education (3rd edition), vol. 7, pp. 112-118. Oxford, UK: Elsevier. PDF Reprint
- Campbell, John P.; Peter B. DeBlois, and Diana G. Oblinger, “Academic Analytics: A New Tool for a New Era,” EDUCAUSE Review, vol. 42, no. 4 (July/August 2007), pp. 40–57, http://www.educause.edu/library/erm0742.
- Dawson, S., Heathcote, L. and Poole, G. (2010), “Harnessing ICT Potential: The Adoption and Analysis of ICT Systems for Enhancing the Student Learning Experience,” The International Journal of Educational Management, 24(2), pp. 116-129.
- Duval, E.; Verbert, K. “On the role of technical standards for learning technologies.” IEEE Transactions on Learning Technologies 1, no. 4 (October 2008): 229-234. https://lirias.kuleuven.be/handle/123456789/234781.
- Dawson, S. (2008). A study of the relationship between student social networks and sense of community, Educational Technology and Society 11(3), pp. 224-38. PDF
- Dawson, ". (2010) Seeing the learning community. An exploration of the development of a resource for monitoring online student networking. British Journal of Educational Technology, 41(5), 736-752. doi:10.1111/j.1467-8535.2009.00970.x.
- Dawson, S., Heathcote, L. and Poole, G. (2010). Harnessing ICT potential: The adoption and analysis of ICT systems for enhancing the student learning experience, International Journal of Educational Management 24(2) pp. 116-128.
- Educause, 2010. 7 Things you should know about analytics, EDUCAUSE 7 things you should know series. Retrieved October 1, 2010 from http://www.educause.edu/ir/library/pdf/ELI7059.pdf
- Ferguson, R. and Buckingham Shum, S. (2012). Social Learning Analytics: Five Approaches. Proc. 2nd International Conference on Learning Analytics & Knowledge, (29 Apr-2 May, Vancouver, BC). ACM Press: New York. Eprint: http://projects.kmi.open.ac.uk/hyperdiscourse/docs/LAK2012-RF-SBS.pdf
- Laat, M. de; V. Lally, L. Lipponen, R. Simons (2007). Investigating patterns of interaction in networked learning and computer-supported collaborative learning: a role for social network analysis, International Journal of Computer-Supported Collaborative Learning, 2 (1), pp. 87–103
Investigating patterns of interaction in networked learning and computer-supported collaborative learning: a role for social network analysis
International Journal of Computer-Supported Collaborative Learning, 2 (1) (14 March 2007), pp. 87–103
- De Liddo, A., Buckingham Shum, S., Quinto, I., Bachler, M. and Cannavacciuolo, L. (2011). Discourse-Centric Learning Analytics. Proc. 1st International Conference on Learning Analytics & Knowledge. Feb. 27-Mar 1, 2011, Banff. ACM Press: New York. Eprint: http://oro.open.ac.uk/25829
- Ferguson, R. and Buckingham Shum, S. (2011). Learning Analytics to Identify Exploratory Dialogue within Synchronous Text Chat. Proc. 1st International Conference on Learning Analytics & Knowledge. Feb. 27-Mar 1, 2011, Banff. ACM Press: New York. Eprint: http://oro.open.ac.uk/28955
- Few, S. (2007). Dashboard Confusion Revisited. Visual Business Intelligence Newsletter. January 2007. PDF
- Goldstein, P. J. (2005) Academic Analytics: Uses of Management Information and Technology in Higher Education PDF
- Goldstein, P. J. and Katz, R. N. (2005). Academic Analytics: The Uses of Management Information and Technology in Higher Education, ECAR Research Study Volume 8. Retrieved October 1, 2010 from http://www.educause.edu/ers0508
- Hadwin, A. F., Nesbit, J. C., Code, J., Jamieson-Noel, D. L., & Winne, P. H. (2007). Examining trace data to explore self-regulated learning. Metacognition and Learning, 2, 107-124
- Haythornthwaite, C. (2008). Learning relations and networks in web-based communities. International Journal of Web Based Communities, 4(2), 140-158.
- Hijon R. and Carlos, R. (2006). E-learning platforms analysis and development of students tracking functionality, in Proceedings of the 18th World Conference on Educational Multimedia,Hypermedia & Telecomunications, pp. 2823-2828.
- Koedinger, K.R., Baker, R.S.J.d., Cunningham, K., Skogsholm, A., Leber, B., Stamper, J. (2010) A Data Repository for the EDM community: The PSLC DataShop. In Romero, C., Ventura, S., Pechenizkiy, M., Baker, R.S.J.d. (Eds.) Handbook of Educational Data Mining. Boca Raton, FL: CRC Press, pp. 43-56.
- Leah P. Macfadyen and Shane Dawson, “Mining LMS Data to Develop an ‘Early Warning System’ for Educators: A Proof of Concept,” Computers & Education, vol. 54, no. 2 (2010), pp. 588–599.
- Libby V. Morris, Catherine Finnegan, and Sz-Shyan Wu, “Tracking Student Behavior, Persistence, and Achievement in Online Courses,” The Internet and Higher Education, vol. 8, no. 3 (2005), pp. 221–231.
- Macfayden, L. P., & Dawson, S. (2010). Mining LMS data to develop an “early warning” system for educators: a proof of concept. Computers & Education, 54(2), 588–599.
- Macfadyen, L. P. and Sorenson. P. (2010) “Using LiMS (the Learner Interaction Monitoring System) to track online learner engagement and evaluate course design.” In Proceedings of the 3rd international conference on educational data mining (pp. 301–302), Pittsburgh, USA.
- Mazza R., and Dimitrova, V. (2004). Visualising student tracking data to support instructors in web-based distance education, 13th International World Wide Web conference, New York, NY, USA: ACM Press, pp. 154-161. PDF, retrieved 16:02, 15 March 2012 (CET).
- Najjar. J; M. Wolpers, and E. Duval. Contextualized attention metadata. D-Lib Magazine, 13(9/10), Sept. 2007.
- Norris, D., Baer, L., Leonard, J., Pugliese, L. and Lefrere, P. (2008). Action Analytics: Measuring and Improving Performance That Matters in Higher Education, EDUCAUSE Review 43(1). Retrieved October 1, 2010 from http://www.educause.edu/EDUCAUSE+Review/EDUCAUSEReviewMagazineVolume43/ActionAnalyticsMeasuringandImp/162422
- Norris, D., Baer, L., Leonard, J., Pugliese, L. and Lefrere, P. (2008). Framing Action Analytics and Putting Them to Work, EDUCAUSE Review 43(1). Retrieved October 1, 2010 from http://www.educause.edu/EDUCAUSE+Review/EDUCAUSEReviewMagazineVolume43/FramingActionAnalyticsandPutti/162423
- Oblinger, D. G. and Campbell, J. P. (2007). Academic Analytics, EDUCAUSE White Paper.Retrieved October 1, 2010 from http://www.educause.edu/ir/library/pdf/PUB6101.pdf
- Rath, A. S., D. Devaurs, and S. Lindstaedt. UICO: an ontology-based user interaction context model for automatic task detection on the computer desktop. In Proceedings of the 1st Workshop on Context, Information and Ontologies, CIAO ’09, pages 8:1—-8:10, New York, NY, USA, 2009. ACM.
- Romero, C. Ventura, S. (in press) Educational Data Mining: A Review of the State-of-the-Art. IEEE Transaction on Systems, Man, and Cybernetics, Part C: Applications and Reviews. PDF
- Siemens George; Dragan Gasevic, Caroline Haythornthwaite, Shane Dawson, Simon Buckingham Shum, Rebecca Ferguson, Erik Duval, Katrien Verbert and Ryan S. J. d. Baker (2001). Open Learning Analytics: an integrated & modularized platform Proposal to design, implement and evaluate an open platform to integrate heterogeneous learning analytics techniques, Society for Learning Analytics Research. PDF, retrieved 22:02, 1 March 2012 (CET).
- Siemens, G., Long, P. (2011). Penetrating the Fog: Analytics in learning and education. EDUCAUSE Review, vol. 46, no. 4 (July/August 2011)
- Siemens, G. (2007) “Connectivism: Creating a Learning Ecology in Distributed Environment,” in Didactics of Microlearning: Concepts, discourses, and examples, in T. Hug, (ed.),Waxmann Verlag, New York, pp. 53-68
- Ternier, S.; K. Verbert, G. Parra, B. Vandeputte, J. Klerkx, E. Duval, V. Ordonez, and X. Ochoa. The Ariadne Infrastructure for Managing and Storing Metadata. IEEE Internet Computing, 13(4):18–25, July 2009.
- Wolpers. M; J. Najjar, K. Verbert, and E. Duval. Tracking actual usage: the attention metadata approach. Educational Technology and Society, 10(3):106–121, 2007.
- Zhang, H., Almeroth, K., Knight, A., Bulger, M., and Mayer, R. (2007). Moodog: Tracking Students' Online Learning Activities in C. Montgomerie and J. Seale (Eds.), Proceedings of World Conference on Educational Multimedia, Hypermedia and Telecommunications, Chesapeake, VA: AACE, 2007, pp. 44154422. DOI 10.1.1.82.951
- Zhang, H. and Almeroth, K. (2010). Moodog: Tracking Student Activity in Online Course Management Systems. Journal of Interactive Learning Research, 21(3), 407-429. Chesapeake, VA: AACE. Retrieved October 5, 2010 from http://www.editlib.org.aupac.lib.athabascau.ca/p/32307.
Planned:
- The Journal of Educational Technology and Society Special Issue on Learning Analytics due in 2012.
- American Behavioral Scientist Special Issue on Learning Analytics due in 2012.
CSCL literature
- Constantino-Gonzalez, M.A., Suthers, D.D. and Escamilla de los Santos, J.G. (2002). Coaching web-based collaborative learning based on problem solution differences and participation. International Journal of Artificial Intelligence in Education, 13, 263-299.
- Dönmez, P., Rosé, C. P., Stegmann, K., Weinberger, A., & Fischer, F. (2005). Supporting CSCL with Automatic Corpus Analysis Technology. In T. Koschmann & D. Suthers & T. W. Chan (Eds.), Proceedings of the International Conference on Computer Support for Collaborative Learning (pp. 125-134). Taipeh, Taiwan.
- Fischer, F., Bruhn, J., Gräsel, C. & Mandl, H. (2002). Fostering collaborative knowledge construction with visualization tools. Learning and Instruction, 12, 213-232.
- Hutchins (1995). How a cockpit remembers its speeds. Cognitive Science, 19, 265-288.
- Jermann, P., Soller, A., & Muehlenbrock, M. (2001). From Mirroring to Guiding: A Review of State of the Art Technology for Supporting Collaborative Learning. Proceedings of the First European Conference on Computer-Supported Collaborative Learning, Maastricht, The Netherlands, 324-331.
- Kolodner, J., & Guzdial, M. (1996). Effects with and of CSCL: Tracking learning in a new paradigm. In T. Koschmann (Ed.) CSCL: Theory and Practice of an Emerging Paradigm (pp. 307-320). Mahwah NJ: Lawrence Erlbaum Associates.
- Mühlenbrock, M., & Hoppe, U. (1999). Computer supported interaction analysis of group problem solving. In C. Hoadley & J. Roschelle (Eds.) Proceedings of the Conference on Computer Supported Collaborative Learning (CSCL-99) (pp. 398-405). Mahwah, NJ: Erlbaum.
- Soller, A., & Lesgold, A. (2003). A computational approach to analyzing online knowledge sharing interaction. Proceedings of Artificial Intelligence in Education 2003, Sydney, Australia, 253-260.
- Soller, Amy, Alejandra Martinez, Patrick Jermann, and Martin Muehlenbrock (2005). From Mirroring to Guiding: A Review of State of the Art Technology for Supporting Collaborative Learning. Int. J. Artif. Intell. Ed. 15, 4 (December 2005), 261-290. ACM digital library. A prior version is available as ITS 2004 Workshop on Computational Models of Collaborative Learning paper
- Soller et al. (eds.) (2004). The 2nd International Workshop on Designing Computational Models of Collaborative Learning Interaction PDF
- Stegmann, K., & Fischer, F. (2011). Quantifying Qualities in Collaborative Knowledge Construction: The Analysis of Online Discussions. Analyzing Interactions in CSCL, 247–268. Springer.
- Wang Yi-Chia, Cui, Y., Arguello, J., Stegmann, K., Weinberger, A., Fischer, F., & Rosé, C. P. (2008). Analyzing collaborative learning processes automatically: Exploiting the advances of computational linguistics in computer-supported collaborative learning. International Journal of Computer-Supported Collaborative Learning, 3(3), 237–271. doi: 10.1007/s11412-007-9034-0.
Acknowledgements and copyright modification
This article is licensed under the Creative Commons Attribution-NonCommercial 3.0 License and you also must cite cite Penetrating the Fog: Analytics in Learning and Education if you reuse parts from that source, e.g. the figure.