Learning analytics
Introduction
One could define learning analytics as collection of methods that allow teachers and maybe the learners to understand what is going on. I.e. all sorts of tools that allow to gain insight on participant's behavior and productions.
The Society for Learning Analytics Research Open Learning Analytics (2011) proposal associates learning analytics with the kind of "big data" that are used in busines intelligence:
The rapid development of “big data” methods and tools coincides with new management and measurement processes in corporations. The term “business intelligence” is used to describe this intersection of data and insight. When applied to the education sector, analytics fall into two broad sectors (Table 1): learning and academic.
Learning analytics (LA) is the measurement, collection, analysis and reporting of data about learners and their contexts, for purposes of understanding and optimizing learning and the environments in which it occurs. Learning analytics are largely concerned with improving learner success.
Academic analytics is the improvement of organizational processes, workflows, resource allocation, and institutional measurement through the use of learner, academic, and institutional data. Academic analytics, akin to business analytics, are concerned with improving organizational effectiveness.In other words, learning analytics concerns people concerned by teaching and learning, e.g. learners themselves, teachers, course designers, course evaluators, etc. Learning analytics is seen {{quotation|LA as a means to provide stakeholders (learners, educators, administrators, and funders) with better information and deep insight into the factors within the learning process that contribute to learner success. Analytics serve to guide decision making about educational reform and learner-level intervention for at-risk students. (Simons et al. 2011: 5)
In that sense, this definition is political and like many other constructs in the education sciences it promises better education. We therefore conclude the introduction that learning analytics either can be seen either (1) as tools that should be integrated into the learning environment and scenario with respect to specific teaching goals or (2) as a more general and bigger "framework" for doing "education intelligence". The latter also would include the former.
Frameworks and technology
Some kinds of learning analytics have been known and used since education education exists like:
- Grades
- learning e-portfolios, i.e. students assemble productions and reflect upon these (use quite a lot in professional education)
- Tracking tools in learning management systems
- Cockpits and scaffolding used in many CSCL tools.
The SoLAR strategy
This proposal addresses the need for integrated toolsets through the development of four specific tools and resources:
- A Learning analytics engine, a versatile framework for collecting and processing data with various analysis modules (depending on the environment)
- Adaptive content engine
- Intervention engine: recommendations, automated support
- Dashboard, reporting, and visualization tools
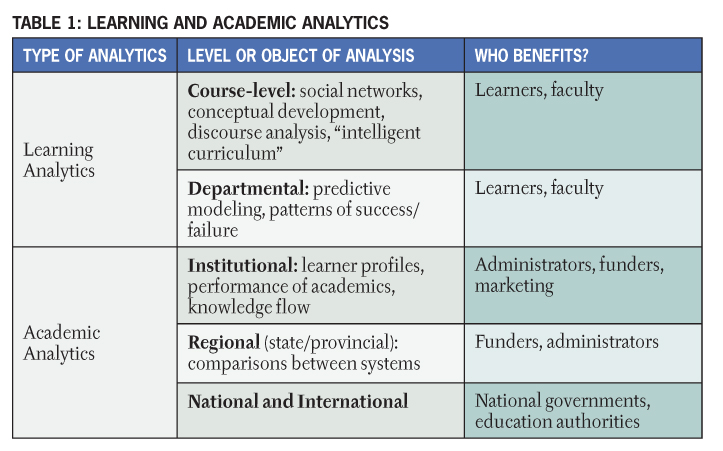
A short discussion
The proposal starts somehow with the assumption that education continues to use rather simple tools like courseware or somewhat now popular web 2.0 tools like personal learning environments. In other words, the fog is identifed as problem and not the way education is designed. I.e. this proposal focuses on "intelligence" as opposed to "design". If you like, is more like "General motors" than "Apple". Related to that it is also assumed that "metrics" work, while in reality testing-crazy systems like the US high-school education has a much lower performance than design-based systems like the Finnish one.
The project proposes a general framework based on modern service-oriented architectures. So far, all attempts to use such architectures in education did fail, probably because of the lack of substantial long-term funding, e.g. see the e-framework project. We also a wonder a bit how such funding could be found, since not even the really simple IMS Learning Design has been implemented in a usable set of tools. In addition, even more simple stuff, like simple wiki tracking is not available, e.g. read wiki metrics, rubrics and collaboration tools
We would like to draw parallels with (1) the metadata community that spent a lot of time designing standards for describing documents and instead of working on how to create more expressive documents and understanding how people compose documents, (2) with business that spends energy on marketing and related business intelligence instead of designing better products, (3) with folks who believe in adaptive systems forgetting that learner control is central to deep learning and that most higher education is collaborative, (4) with the utter failure of intelligent tutoring systems trying to give control to the machine and (5) finally with the illusion of learning style. These negative remarks are not meant to say that this project should or must fail, but they are meant to state two things: The #1 issue is in education is not analytics, but designing good learning scenarios within appropriate learning environements (most are not). The #2 issue is massive long term funding. Such a system won't work before at least 50 man years over a 10 year period is spend.
Somewhat it also is assumed that teachers don't know what is going on and that learners can't keep track of their progress or more precisely that teachers can't design scenarios that will help both teachers and students knowing what is going on. We mostly share that assumption, but would like to point out that knowledge tools do exist, e.g. knowledge forum, but these are never used. This can be said in general of CSCL tools that usually include scaffolding and meta-reflective tools. In other words, this proposal seems to imply that that education and students will remain simple, but "enhanced" with both teacher and student cockpits.
Finally, this project raises deep privacy issues, in particle since analytics can be used for assessment. In addition, if students are require to play the analytics game in order to improve chances, this will be other blow to to creativity (after SCORM-based teaching).
Links
- Organizations
- Society for Learning Analytics Research (SoLAR), “on inter-disciplinary network of leading international researchers who are exploring the role and impact of analytics on teaching, learning, training and development.”
- International Educational Data Mining Society
- Talks
- By Simon Buckingham Shum, jan 2012.
Bibliography
This bibliography is so far based on the Open Learning Analytics proposal and Penetrating the Fog EduCause article.
- Baker, R.S.J.d., de Carvalho, A. M. J. A. (2008) Labeling Student Behavior Faster and More Precisely with Text Replays. Proceedings of the 1st International Conference on Educational Data Mining, 38-47.
- Baker, R.S.J.d., Yacef, K. (2009) The State of Educational Data Mining in 2009: A Review and Future Visions. Journal of Educational Data Mining, 1 (1), 3-17.
- Campbell, John P.; Peter B. DeBlois, and Diana G. Oblinger, “Academic Analytics: A New Tool for a New Era,” EDUCAUSE Review, vol. 42, no. 4 (July/August 2007), pp. 40–57, <http://www.educause.edu/library/erm0742>.
- Dawson, S., Heathcote, L. and Poole, G. (2010), “Harnessing ICT Potential: The Adoption and Analysis of ICT Systems for Enhancing the Student Learning Experience,” The International Journal of Educational Management, 24(2), pp. 116-129.
- Duval, E.; Verbert, K. “On the role of technical standards for learning technologies.” IEEE Transactions on Learning Technologies 1, no. 4 (October 2008): 229-234. https://lirias.kuleuven.be/handle/123456789/234781.
- Ferguson, R. and Buckingham Shum, S. (2012). Social Learning Analytics: Five Approaches. Proc. 2nd International Conference on Learning Analytics & Knowledge, (29 Apr-2 May, Vancouver, BC). ACM Press: New York. Eprint: http://projects.kmi.open.ac.uk/hyperdiscourse/docs/LAK2012-RF-SBS.pdf
- De Liddo, A., Buckingham Shum, S., Quinto, I., Bachler, M. and Cannavacciuolo, L. (2011). Discourse-Centric Learning Analytics. Proc. 1st International Conference on Learning Analytics & Knowledge. Feb. 27-Mar 1, 2011, Banff. ACM Press: New York. Eprint: http://oro.open.ac.uk/25829
- Ferguson, R. and Buckingham Shum, S. (2011). Learning Analytics to Identify Exploratory Dialogue within Synchronous Text Chat. Proc. 1st International Conference on Learning Analytics & Knowledge. Feb. 27-Mar 1, 2011, Banff. ACM Press: New York. Eprint: http://oro.open.ac.uk/28955
- Hadwin, A. F., Nesbit, J. C., Code, J., Jamieson-Noel, D. L., & Winne, P. H. (2007). Examining trace data to explore self-regulated learning. Metacognition and Learning, 2, 107-124
- Haythornthwaite, C. (2008). Learning relations and networks in web-based communities. International Journal of Web Based Communities, 4(2), 140-158.
- Koedinger, K.R., Baker, R.S.J.d., Cunningham, K., Skogsholm, A., Leber, B., Stamper, J. (2010) A Data Repository for the EDM community: The PSLC DataShop. In Romero, C., Ventura, S., Pechenizkiy, M., Baker, R.S.J.d. (Eds.) Handbook of Educational Data Mining. Boca Raton, FL: CRC Press, pp. 43-56.
- Leah P. Macfadyen and Shane Dawson, “Mining LMS Data to Develop an ‘Early Warning System’ for Educators: A Proof of Concept,” Computers & Education, vol. 54, no. 2 (2010), pp. 588–599.
- Libby V. Morris, Catherine Finnegan, and Sz-Shyan Wu, “Tracking Student Behavior, Persistence, and Achievement in Online Courses,” The Internet and Higher Education, vol. 8, no. 3 (2005), pp. 221–231.
- Macfayden, L. P., & Dawson, S. (2010). Mining LMS data to develop an “early warning” system for educators: a proof of concept. Computers & Education, 54(2), 588–599.
- Najjar. J; M. Wolpers, and E. Duval. Contextualized attention metadata. D-Lib Magazine, 13(9/10), Sept. 2007.
- Rath, A. S., D. Devaurs, and S. Lindstaedt. UICO: an ontology-based user interaction context model for automatic task detection on the computer desktop. In Proceedings of the 1st Workshop on Context, Information and Ontologies, CIAO ’09, pages 8:1—-8:10, New York, NY, USA, 2009. ACM.
- Romero, C. Ventura, S. (in press) Educational Data Mining: A Review of the State-of-the-Art. IEEE Transaction on Systems, Man, and Cybernetics, Part C: Applications and Reviews.
- Siemens George; Dragan Gasevic, Caroline Haythornthwaite, Shane Dawson, Simon Buckingham Shum, Rebecca Ferguson, Erik Duval, Katrien Verbert and Ryan S. J. d. Baker (2001). Open Learning Analytics: an integrated & modularized platform Proposal to design, implement and evaluate an open platform to integrate heterogeneous learning analytics techniques, Society for Learning Analytics Research. PDF, retrieved 22:02, 1 March 2012 (CET).
- Siemens, G., Long, P. (2011). Penetrating the Fog: Analytics in learning and education. EDUCAUSE Review, vol. 46, no. 4 (July/August 2011)
- Siemens, G. (2007) “Connectivism: Creating a Learning Ecology in Distributed Environment,” in Didactics of Microlearning: Concepts, discourses, and examples, in T. Hug, (ed.),Waxmann Verlag, New York, pp. 53-68
- Ternier, S.; K. Verbert, G. Parra, B. Vandeputte, J. Klerkx, E. Duval, V. Ordonez, and X. Ochoa. The Ariadne Infrastructure for Managing and Storing Metadata. IEEE Internet Computing, 13(4):18–25, July 2009.
- Wolpers. M; J. Najjar, K. Verbert, and E. Duval. Tracking actual usage: the attention metadata approach. Educational Technology and Society, 10(3):106–121, 2007.
Planned:
- The Journal of Educational Technology and Society Special Issue on Learning Analytics due in 2012.
- American Behavioral Scientist Special Issue on Learning Analytics due in 2012.
Acknowledgements and copyright modification
This article is licensed under the Creative Commons Attribution-NonCommercial 3.0 License and you also must cite cite Penetrating the Fog: Analytics in Learning and Education if you reuse parts from this source, e.g. figures and the bibliography.